Unlocking the Human Value of Machine Learning
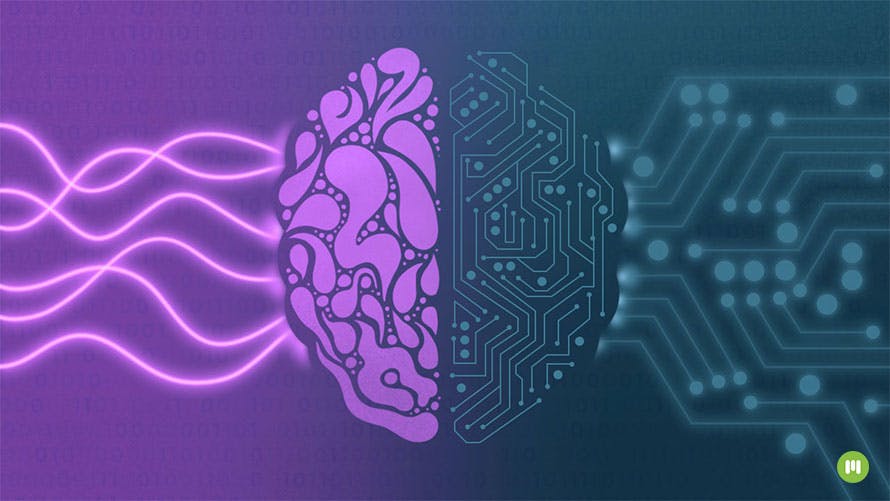
Data, Data Everywhere
Our world is overwhelmed by data. Last year, humanity created an average of 1.7 MB of data per person per day. That's 2.5 quintillion new data bytes in 2020, bringing the total digital universe to an almost incomprehensible 44 zettabytes. Since millions of new people join the wired world every year while the existing population creates ever-more data, that number will only continue to expand.
The future is data. And data has the potential to transform the future, but only if it's possible to separate the signal from the noise. (With so much data, there's a lot of noise!) Machine learning (ML) can be a core piece of digital business transformation.
When used effectively, ML sifts through vast quantities of information, revealing transformative insights and innovations that wouldn't be possible to recognize otherwise. Business leaders are catching on to the promise with 83 percent and 69 percent of IT leaders reporting that artificial intelligence and machine learning is transforming their customer engagement and business, respectively, according to a Salesforce survey. As a result, the ML market is projected to grow from $7.3 billion in 2020 to $30.6 billion in just four years.
That's the good news. The bad? Doing machine learning correctly is difficult. Almost nine out of every 10 ML projects never make it into production. Rather than delivering smart, sophisticated, and actionable business solutions, these efforts stay metaphorically mothballed, their only legacy one of good intentions and wasted effort.
But it doesn't have to be this way. Smart business leaders know that machine learning isn't the end point; instead, it's a tool. Too many people simply want to say they are using ML when the reality is that merely using it isn't enough. Successful projects will have clear, actionable goals, success metrics, and—this is the key—a focus on the people who will be seeing the results. When it comes to ML, it's far too easy to overlook the human element. Doing so is a mistake.
Goals Before Growth
You wouldn't build a company or consider launching a new product without a roadmap of where you're going and how you're going to get there. That map will certainly change along the way as not even the best business leaders can predict the future—and flexibility is a key to achieving goals—but having one at the outset is an essential element of achieving success.
The same idea goes for any ML project. At the beginning, ask yourself and your team a few questions.
What are you trying to accomplish? What pain point are you addressing and solving? Is that something machine learning can help? Then, delve down a bit deeper and think about data. What type of data will you be able to gather? Will it be good, clean data that's easy to manipulate with machine learning? Without the right type of quality data, any ML project will fail.
When designing a machine learning exercise or product, technologists need to consider what will be helpful data, have a plan to gather it, and build based on this expected information. Think twice and build once. “The goal should be to have the machine learning model take the first pass through these mountains of data and draw relevant insights out,” Modus Agency CEO Rick Levine says. “Success is a combination of uncovering hidden learnings and presenting that information in an interesting way so it grabs the user’s attention.”
It's better to start with a simple model that works and gradually increase the level of sophistication than begin with something overly complex that may or may not accomplish the task you hope.
ABI (Always Be Iterating)
You have a model and it's producing results. Congrats. That's quite an accomplishment, one that many businesses never realize with their machine learning attempts. But don't stop now. Keep pushing. Successful ML requires constant monitoring of what is working and what is not, then refining and tweaking the model as necessary. Don't be afraid to be flexible. The KPIs you established at the beginning of the project might need to change as you learn what your model can and cannot achieve.
Experiment, test, iterate, repeat.
You might even need to pivot completely. Remember, machine learning models are not an end in and of themselves. Instead, they are a tool to solve business solutions and deliver real-world value. The most elegant and creative ML project isn't useful if it doesn't drive change or create useful insight. If a path is leading to a dead end, don't try to find a way through. Instead, return to an earlier stage and go in a different direction entirely.
The Human Element
Too often, the human gets forgotten when the machines get involved. This is a mistake, and leads to outcomes where machine learning models are tailored to issues that aren't actually relevant to the users. Never forget that the goal is to help people, to design a system that's easy to use, easy to understand, and has useful outcomes.
Take how Airbnb developed an ML model that identifies and classifies guest messages, which can “greatly shorten the response time for guests and reduce the overall workload required for hosts.” This idea is simple—nothing more than automatically handling some easy interactions between two parties—but developing the ML model requires thinking about how real people use the platform and solving for their needs.
Companies including Facebook, Amazon, and Netflix are also using similar technology. Consider how they just seem to know what you want to see, buy, or watch, and how the interface works to reinforce this message. “The key is to take the data and figure out how to show it to the end user so that they can make value out of it,” Levine says. “We’re providing the information that they want so that they're going to be able to take action on what's most valuable to them.”
ML and the Future
Value created by unlocking mountains of data is here to stay. So is ML. The successful enterprises of the future will capitalize on the efficiencies created while also prioritizing the outputs from a design perspective. Machine learning needs to create value for businesses, which really means it needs to create value for the people who are a part of those businesses. Without engineering and designing for that outcome, and keeping the human element front of mind when testing, tweaking, updating, and iterating, ML models will fall short of their enormous potential.